AI in Remote Patient Monitoring: Use Cases, Challenges, Implementation
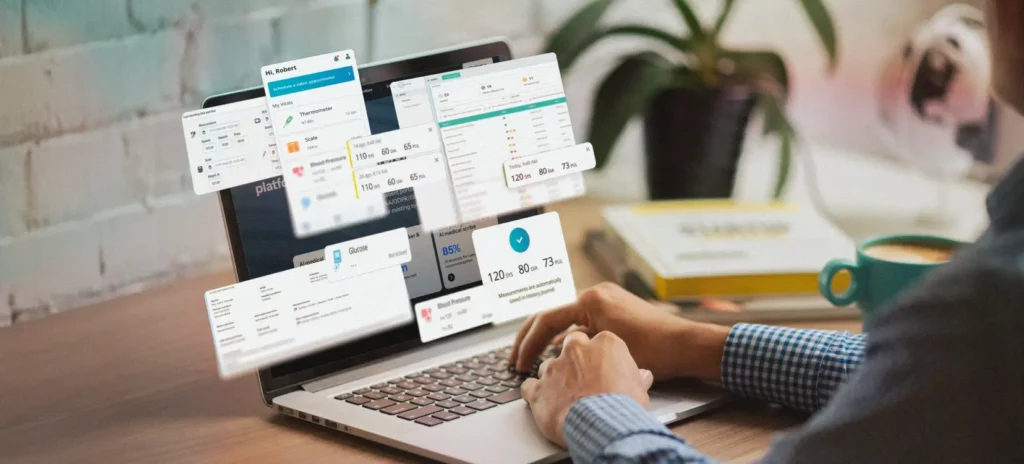
AI in remote patient monitoring is transforming patient care from afar, and it’s a win-win for everyone. Imagine a healthcare system under strain—overflowing hospitals, endless waitlists, and the growing burden of chronic disease. This is today’s reality.
To meet these challenges, healthcare must shift from reactive to proactive care. That’s where Artificial Intelligence (AI) in remote patient monitoring (RPM) comes in. RPM tracks patients’ vital signs outside the hospital, and with AI, it evolves into a predictive, personalized, and scalable solution.
This article explores how AI-driven RPM works, where it brings the most value, the challenges to implementation, and why partners like Riseapps are helping best healthcare companies shape the future of patient monitoring.
What is AI patient monitoring?
RPM involves collecting health data from patients in non-clinical settings (home, workplace, etc.) using sensors, wearables, and mobile apps. This historical patient data typically includes heart rate, blood pressure, oxygen saturation, glucose levels, activity levels, sleep, and medication adherence.
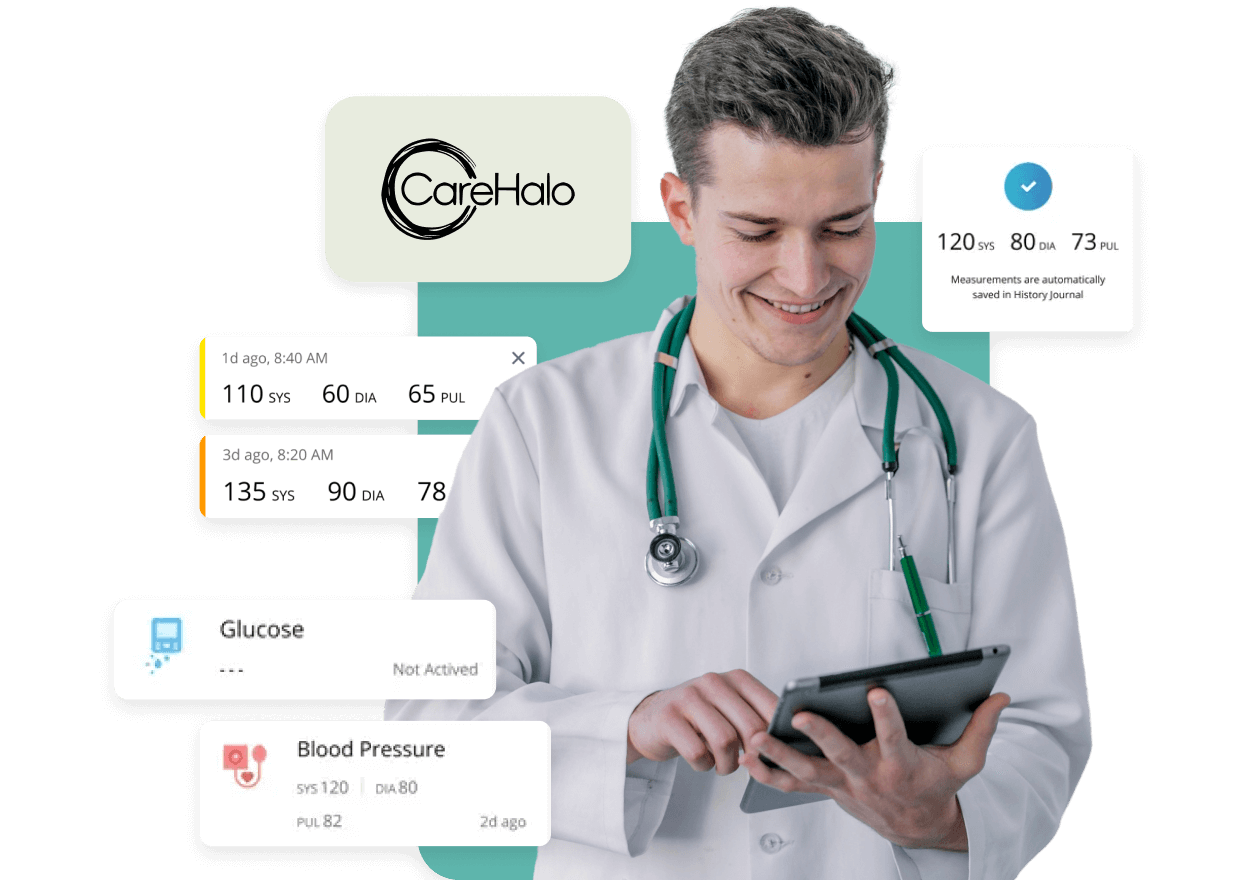
Monitoring systems are set up to trigger alarms if these vital signs go outside the normal range, so medical staff can spot a health crisis right away and get involved straight away.
At Riseapps, we have developed an advanced dementia care platform that tracks and manages patients’ vital signs in real time, helping improve patient outcomes and caregiver support.
The integration of AI into Remote Patient Monitoring transcends the traditional limitations of simple data collection, transforming it into a dynamic, predictive, and personalized healthcare tool. AI in patient monitoring brings intelligence to RPM systems. It interprets data using:
- Machine Learning (ML) – learns from historical data to predict trends
- Natural Language Processing (NLP) – analyzes patient-reported symptoms or journaling
- Computer Vision – interprets images or video (e.g., wound healing, movement patterns)
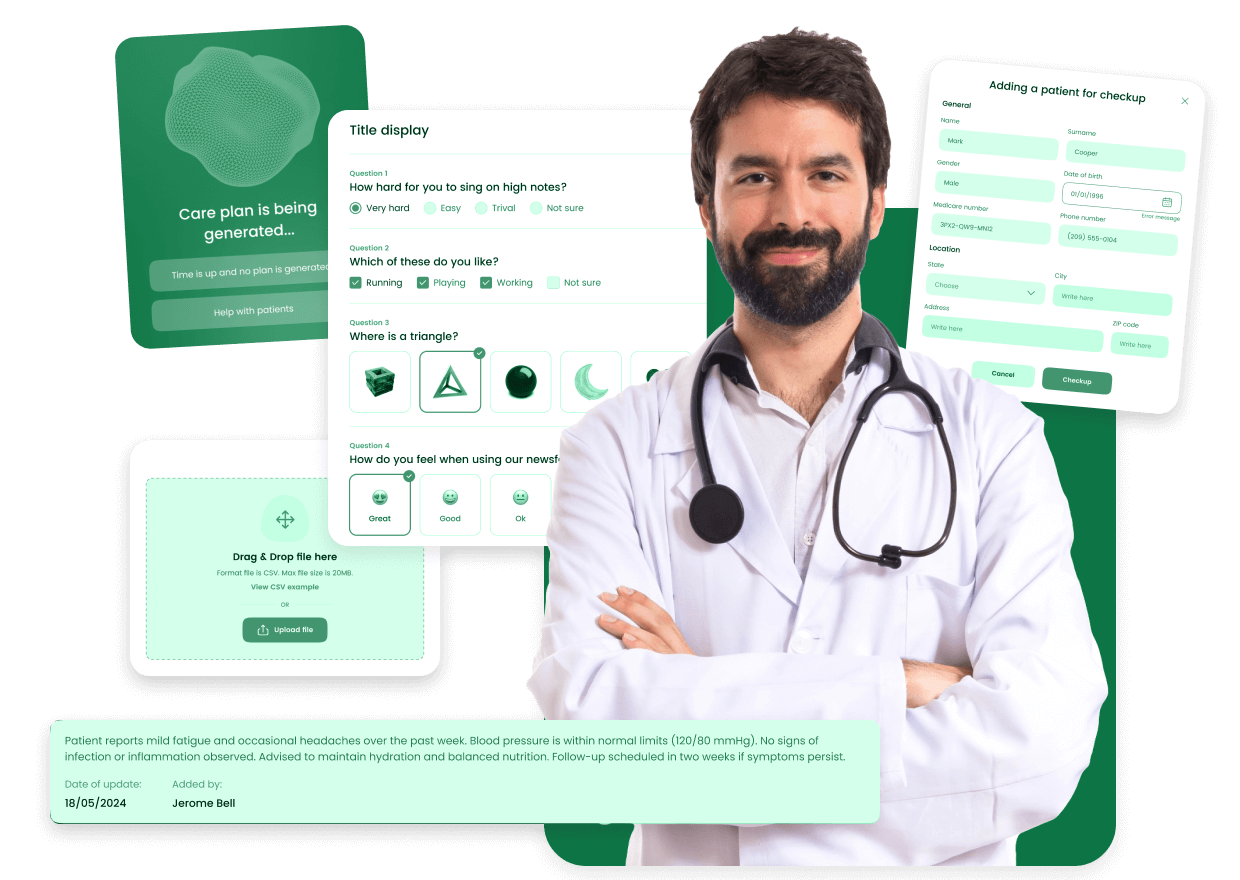
AI-powered wearables and sensors provide continuous streams of data, which are then analyzed by machine learning algorithms to detect anomalies and trends, enabling healthcare providers to intervene proactively. Ultimately, the integration of AI into RPM enables proactive health interventions, early diagnosis, and disease prevention, leading to improved patient outcomes and more efficient healthcare delivery.
The main goal of AI in patient monitoring is to transition from reactive care (responding to symptoms) to proactive care—predicting adverse events before they happen and tailoring care to individual patient needs.
The transformative role of AI in remote patient monitoring
The integration of artificial intelligence with wearable sensors and medical devices is revolutionizing remote patient monitoring. Here’s how:
Detecting what humans can’t: subtle patterns and meaningful signals
AI excels at identifying subtle patterns and correlations in health data that are often invisible to the human eye. By filtering out “data noise,” AI models highlight clinically relevant information and reduce information overload.
Example:
Let’s take a look at practical case of AI in remote patient monitoring: a scenario of early detection of Heart Failure (HF) decompensation.
A patient with chronic heart failure is discharged home after a hospitalization. They are enrolled in a remote patient monitoring program.
Data collected remotely:
- Daily weight, using a smart scale.
- Blood pressure & heart rate, using a Bluetooth cuff.
- Oxygen saturation (SpO2), using a pulse oximeter.
- Activity levels, using a wearable fitness tracker (steps, active minutes).
- Patient-reported symptoms via a simple daily check-in app (e.g., rating shortness of breath, fatigue, swelling on a scale).
- Heart Rate Variability (HRV), measured by wearables during rest/sleep.
The challenge for humans:
A nurse reviewing this data daily might see slight variations: weight fluctuating by 0.5-1 lb day-to-day, blood pressure slightly higher some days, activity levels varying based on how the patient “feels.” It’s hard for a human reviewer, looking at incoming data from potentially dozens of high-risk patients, to consistently integrate subtle, noisy data points and spot a developing trend.
How AI excels in detecting subtle patterns:
The AI model, trained on vast amounts of historical HF patient data, can analyze these multiple streams simultaneously over time and detect subtle patterns days before obvious clinical signs appear. It might identify a combination of a gradual upward trend in daily weight, a slight but steady decrease in average daily step count, a subtle increase in resting heart rate, particularly overnight, and distinguish these trends from random daily fluctuations.
Practical outcome:
Based on collected data, the AI sends an alert for the clinical team: “Patient shows a high probability of early decompensation.” The nurse contacts the patient. Instead of waiting for severe symptoms, they can proactively intervene and avert a decompensation crisis, avoid an emergency room visit or hospitalization, help the patient, and reduce healthcare costs.
Predictive analytics & early alerts
Traditional monitoring relies on rigid thresholds, but AI enables more dynamic and predictive care:
- Beyond static alerts: AI-powered systems forecast potential adverse events like heart failure exacerbation, diabetic ketoacidosis, or sepsis before they become critical.
- Risk stratification: Patients are grouped into low-, medium-, and high-risk tiers based on data trends, allowing clinicians to prioritize interventions and allocate resources efficiently.
- Timely action: With early warning, healthcare teams can act on minimal deviations from a patient’s baseline, often preventing hospitalization altogether.
Personalized care at scale
AI doesn’t treat everyone the same—and that’s its strength. It tailors monitoring parameters, alert thresholds, and treatment recommendations to each individual’s profile (a rare possibility when it comes to remote patient care):
- Personalized monitoring: Parameters adjust based on patient history, demographics, and prior responses to treatment.
- Dynamic interventions: As AI in remote patient monitoring continuously analyzes real-time and historical data, it recommends specific personalized treatment plans: medication tweaks, lifestyle adjustments, or scheduling of consultations.
Diagnostic support (not replacement)
A common question is: Can AI diagnose patients? While AI doesn’t replace clinical judgment, it significantly supports diagnostics with collected data.
Example:
A prime case of AI in remote patient monitoring is AliveCor KardiaMobile is an FDA-cleared, pocket-sized personal ECG device. Users place their fingers on two sensors, and it records a medical-grade single-lead ECG on their smartphone via an app.
Use case:
The AI can automatically detect several types of heart conditions. A patient with known paroxysmal (intermittent) AFib uses KardiaMobile daily or when they feel symptoms. One morning, they feel fine but take a routine reading. The AI analyzes the ECG and flags it as “Possible Atrial Fibrillation.” The user send this ECG strip to their cardiologist.
The cardiologist receives the AI-flagged ECG. They review the strip visually to confirm the AI’s finding. Knowing that the patient is currently in AFib, even without symptoms, they make treatment decisions – adjust medication dosage, decide on the timing for a cardioversion procedure, or simply confirm the presence of the arrhythmia.
Medication adherence and engagement
Treatment plans only work if patients follow them. AI helps close this gap by:
- Predicting nonadherence: Based on current health status, patterns in behavior or missed readings, AI can predict when a patient might skip medication.
- Proactive reminders: Patients receive tailored notifications via apps, SMS, or chatbot reminders. This way AI empowers patients to stay on top of their game in avoiding potential health complications.
- Motivational feedback: Remote patient monitoring and AI-generated messages reinforce the importance of treatment, improving engagement and patient outcomes.
Automating what doesn’t require a human
Many clinical tasks are repetitive but necessary. AI lightens the load for healthcare providers:
- Report generation: Automatically summarizes patient data into easy-to-read clinical summaries.
- Triage and prioritization: AI assigns urgency levels to alerts, preventing alert fatigue and ensuring fast response to high-risk cases.
- Admin efficiency: From scheduling and billing to voice transcription, AI streamlines back-office operations.
Example:
Riseapps developed an automated summarization platform using AI algorithms to process and organize electronic health records. Leveraging AWS and Amazon Comprehend Medical allowed better scalability and ReactSignalR enabled real-time updates.
Empowering clinicians through training
AI isn’t for patients only—it also strengthens the healthcare workforce:
- Smart training modules: AI-based simulations and interactive modules help staff master RPM systems quickly.
- Ongoing learning: AI adapts training based on clinician knowledge gaps, roles, and performance feedback.
- AR/VR simulations: Enable remote, hands-on learning experiences to build diagnostic or data interpretation skills.
5 key use cases for AI-driven remote patient monitoring
1. Chronic disease management
AI empowers proactive care for chronic conditions like heart failure, diabetes, and COPD by analyzing multi-source data in real time—vital signs, activity levels, patient-reported outcomes—to detect early signs of deterioration and improve patient outcomes.
Predictive models track indicators such as blood pressure, respiratory rate, oxygen saturation, blood glucose and predicts flare-ups before they become crises.
Real-world examples: The FDA-cleared HeartLogic™ platform by Boston Scientific predicts heart failure decompensation up to 34 days in advance.
Riseapps case study: CareHalo remote patient monitoring (RPM) module is a critical part of their larger chronic care platform. It tracks and manages patients’ vital signs in real time, creating an efficient, scalable workflow for medical staff.
2. Post-operative & transplant patient monitoring
AI extends clinical oversight beyond hospital walls, enabling earlier detection of complications in recovery phases after surgeries or organ transplants.
A cardiac surgery patient recovering at home is monitored via a wearable and smart thermometer. Though each sign alone is subtle, the AI interprets them collectively as early signs of infection and prompts a remote consultation, leading to early treatment.
Real-world example: Current Health’s remote care platform uses AI for remote patient monitoring of post-surgical patients to detect early complications, reducing readmission rates.
3. Fall detection and prevention in the elderly
Falls are a leading cause of injury and death among older adults. AI in remote patient monitoring offers not only detection but prediction through continuous, context-aware observation.
Real-world example: SafelyYou uses AI-powered cameras in memory care facilities to detect and predict falls, reducing fall-related ER visits by 80%.
4. Medication adherence and management
Non-adherence to medication is a widespread challenge for healthcare industry with significant clinical and economic implications. AI enables smart medication support systems that combine real-time tracking with context-aware insights.
Real-world example: MedMinder’s smart pill dispensers use AI to detect non-compliance trends and alert caregivers, healthcare providers or clinicians for intervention.
5. Mental health monitoring
AI brings a new layer of objectivity and responsiveness to behavioral health, particularly where stigma or access issues delay care.
Natural language processing tools analyze text interactions—such as chatbot conversations or journal entries—for changes in sentiment or tone. Meanwhile, an AI chatbot notices increased negative sentiment in patient’s daily journal entries. The system recommends a check-in with the patient’s therapist, enabling earlier intervention and improving patient outcomes.
Real-world example: Woebot Health uses AI-powered conversational agents, patient data and sentiment analysis to monitor mental well-being and provide CBT-based support.
How to overcome the challenges in AI-powered RPM implementation
While AI has the potential to revolutionize remote patient monitoring, its real-world deployment via healthcare systems faces a few challenges:
Data privacy and security
AI-powered RPM solutions handle highly sensitive Protected Health Information, making data privacy and security a top priority.
- Strict compliance requirements.
- High risk of data breaches.
- Critical level of vendor responsibility.
Data quality, integration, and bias
RPM data comes from a growing ecosystem of devices, from wearables to smart home sensors that remote patient uses. AI can only be as accurate as the data it receives.
- Device data must be accurate and standardized.
- Interoperability is essential.
- Bias in training data can reinforce health inequities.
Regulatory landscape and validation
Introducing AI into clinical care means entering a complex and vivid regulatory environment for healthcare providers to navigate. AI algorithms with diagnostic or therapeutic impact are regulated as Software as a Medical Device (SaMD). This requires FDA or equivalent approval, which involves significant documentation and testing.
- Clinical validation is non-negotiable.
- Evolving standards of healthcare industry add uncertainty.
Cost and infrastructure
The promise of AI remote patient monitoring doesn’t come cheap, especially when deployed in a clinical-grade context.
- Initial costs are substantial.
- Robust security measures come with a price tag.
- Operational costs persist. Ongoing maintenance, patient data performance tuning, model retraining, compliance updates, and clinician support all require time and budget.
- Scalability needs to be built in from the start.
Clinician adoption and trust
For AI to support, and not hinder, clinical decision-making, medical professionals and providers need to trust and understand how it works.
The “black box” problem fuels skepticism. Many clinicians are hesitant to rely on AI systems that don’t explain their reasoning.
Overall, 35% of physicians reported that their enthusiasm for health AI exceeded their concerns, up from 30% who felt that way a year earlier. Those who said their concerns exceeded their enthusiasm for the technology dropped to 25% in 2024, down from 29% in 2023. Physicians who were equally excited and concerned remained about the same—with two in five physicians feeling that way.
“The AMA survey illustrates that physicians are increasingly intrigued by the assistive role of health AI and the potential of AI-enable tools to reduce administrative burdens, enhance diagnostic accuracy, and personalize treatments,” said AMA Immediate Past President Jesse M. Ehrenfeld, MD, MPH.
Patient acceptance and digital equity
AI-driven RPM requires patient participation—but not all patients are equally equipped or willing to engage. in personalized treatment plans
Older adults or those in rural/low-income communities may struggle with app-based tools, wearable devices, or Wi-Fi–dependent systems.
Access is a serious issue. Patients need onboarding, coaching, and technical help to stay engaged and compliant over time.
Future trends in AI-Driven patient monitoring
With the evolution of the AI-driven healthcare, here are some key trends that will further enhance the RPM:
Hyper-personalization
One of the most exciting trends in AI-driven RPM is hyper-personalization, which leverages the concept of digital twins, where AI/machine learning models simulate individual’s physiological processes and how they respond to various stimuli (medications, activity, diet, stress, illness).
Edge AI
Edge AI refers to performing data analysis directly on the device, rather than sending it to a remote server for processing. This helps protect sensitive health data while working with real time health metrics.
Explainable AI
Explainable AI in remote patient monitoring ensures that healthcare providers can understand how and why certain conclusions or recommendations are made by an algorithm, fostering trust and confidence.
Multimodal integration
The future of RPM involves the integration of multimodal data to create a holistic view of a patient’s health. This includes not only traditional health data but also genetic information, social determinants of health and behavioral health data.
Federated learning
As AI systems become more sophisticated, Federated Learning will play a role in patient data privacy and security. This approach ensures that sensitive patient information remains within local devices or systems.
Why Riseapps for your AI-driven remote patient monitoring needs?
Here’s why Riseapps is uniquely positioned to help you unlock the full potential of AI in patient monitoring:
- We have extensive experience in building healthcare applications. Our commitment to privacy and industry knowledge enable us to build AI-powered RPM systems that are secure, efficient, and meet the requirements of regulatory compliance.
- Riseapps has a proven track record in healthcare app development services with AI and ML expertise, including the creation of advanced predictive modeling, data analysis tools, and pattern recognition algorithms.
- We specialize in integrating diverse healthcare data sources to build cohesive AI-driven RPM systems. We also ensure these solutions are scalable, and able to evolve alongside your organization’s needs.
- We make custom solutions for patient care, knowing that every healthcare provider and patient population has unique needs.
- Riseapps has a proven track record in delivering high-impact projects in the healthcare space that protect sensitive health information and patient data collected, while improving future health outcomes.
AI in patient monitoring is more than a tech trend. It has arrived to become a transformative force in healthcare. By enabling proactive care, personalized treatments, and real-time insights, AI for remote patient monitoring helps healthcare systems move beyond crisis mode toward sustainable, value-based care.
Do you need to deploy AI RPM that will be scalable, secure, and future-ready to grow with your business? Contact our managers at Riseapps today.
FAQ
How is AI used in patient monitoring?
AI enhances patient monitoring through advanced data analysis, pattern recognition, and predictive alerts.
What is an AI monitoring system?
An AI monitoring system refers to a technology platform that uses artificial intelligence to continuously track and analyze patient data from wearables, sensors, and other health tracking devices. I
Can AI be used to diagnose patients?
AI assists clinicians in diagnosing patients by analyzing data, recognizing patterns, and highlighting potential issues. However, diagnosis is ultimately a clinical judgment made by healthcare professionals.
Is AI used in health tracking?
Yes, AI is commonly used in health tracking systems. Common use cases are continuous monitoring, promoting medication adherence, and averting disease related complications. AI improves patient safety and reduces adverse outcomes.
What role does AI play in remote patient monitoring?
In RPM, AI plays a pivotal role by enhancing data analysis, enabling predictive analytics, and personalizing care plans. Early detection of symptoms and chronic disease management help avoid adverse health events and improve treatment outcomes.
What are the benefits of AI in remote patient monitoring?
The benefits of AI in RPM include: early intervention, fewer hospitalizations, better treatment outcomes, efficient workflows, managing chronic diseases and offsetting disease progression, and personalized care.
How can Riseapps help implement AI in RPM?
Riseapps has a proven track record of delivering custom AI solutions for remote patient monitoring. We are experienced in integrating AI into existing healthcare systems, analyzing data you have at hand, all while helping stakeholders avoid increased healthcare costs. Our team is committed to ensuring HIPAA compliance and using the latest AI/ML technologies to optimize patient care.
Contact Us
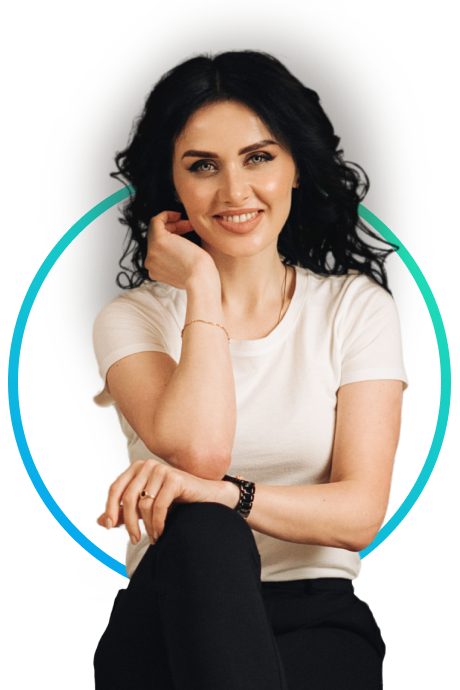