How AI Predictive Analytics in Healthcare Reshapes the Field
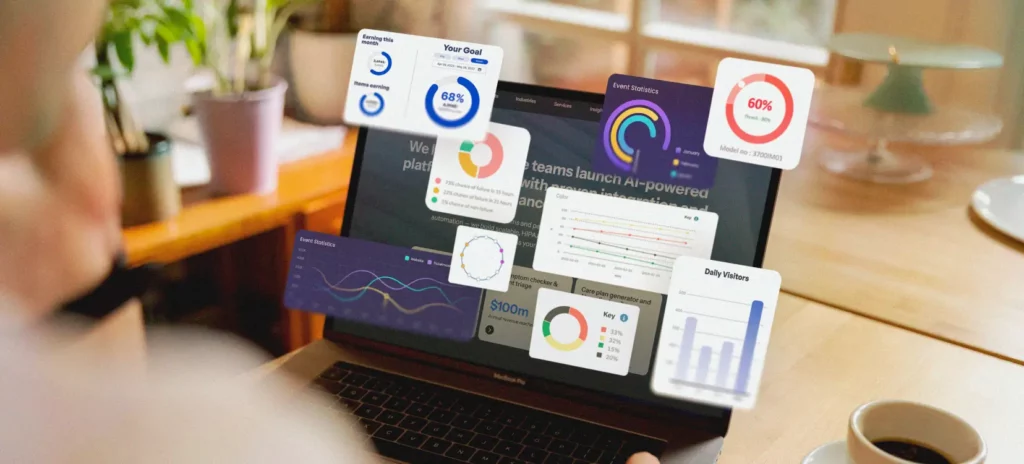
Did you know that life expectancy has more than doubled in the last 100 years, from an average of 32 years in 1900 to 71 years in 2023? It is growing exponentially thanks to the medical innovations, and AI predictive analytics in healthcare is promising to drive another growth spurt for the healthy and longevous humanity.
Thanks to real-world cases, which will be described further in the article, it becomes clear today – AI in general and AI predictive healthcare analytics in particular are changing patient care forever.
AI predictive analytics in healthcare: challenges and the opportunities that arise
Modern healthcare struggles with ensuring patient safety and providing optimal care. Key challenges include fragmented data, cybersecurity risks, medication errors, and post-operative complications. Improving triage processes, early disease detection, and maternal care are essential, alongside streamlining administrative tasks and resolving the ongoing staff shortage crisis to enhance overall efficiency and patient outcomes.
Analyzing healthcare utilization can help identify trends and outcomes, providing insights into how resources are used and where improvements can be made.
The introduction of AI predictive analytics in healthcare helps to solve these pressing problems.
➡️ See Riseapps AI predictive analytics case: BlackDoctor 24/7 app.
AI medtech market development
Almost all the main artificial intelligence technologies are now being applied in the real practice of healthcare organizations, improving the quality of medical services and increasing the duration and quality of life of citizens. Intelligent technologies help predict the occurrence and development of diseases, detect them at an early stage, which increases the chance of successful treatment.
As Precedence Research states, in 2025, the global AI market in healthcare is estimated at 36.96 billion US dollars, and by 2034 it is projected to reach approximately 613.81 billion US dollars, increasing by an average of 36.83% between 2025 and 2034.
At the same time, according to Markets and Markets, 85% of global healthcare leaders are already investing or planning to invest in this technology over the next three years. This trend will accelerate in 2025 as the industry strives to improve workflow efficiency.
Predictive analytics solutions are driving this growth by providing advanced tools for disease prediction and patient care optimization.
What is AI predictive analytics in healthcare?
AI predictive analytics is a complex early warning system that uses your historical data (patient records, operational information) combined with artificial intelligence to identify patterns and predict:
- Which patients are at risk?
- When you will need more beds?
- What potential diagnoses to prepare for?
AI predictive analytics is based on the answer to the question: “What can happen?” It is a transition from reaction to proactive preparation. AI also eliminates the need for clinical trials due to the possibilities of virtual simulation.
According to the article by Svechkareva I.R., Gusev A.V., Kolbin A.S. “Prospects of AI in preclinical and clinical research. Clinical Pharmacology and Therapy” (2025), AI makes the drug development process more cost-effective already.
Healthcare predictive analytics examines patient data to find patterns and predict patient outcomes. It collects data from electronic medical records, wearable devices, and genetic data. AI tools help doctors notice what they might have missed. This leads to faster detection of outbreaks and better containment.
The Canadian AI company BlueDot is a great example of predictive analytics and AI solutions in healthcare. Namely, it predicted the outbreak of COVID-19 by analyzing airline data, news, and disease reports.
Thus, AI helps healthcare organizations respond quickly to outbreaks, improving health outcomes.
Benefits of AI predictive analytics in healthcare
Here are just a few of predictive analysis in healthcare benefits as seen in real-world use cases.
Cardiology
AI tools in cardiology analyze heart images, ECG waveforms, and laboratory tests to detect cardiovascular diseases and manage chronic diseases.
Ultromics
It has developed the EchoGo platform, which helps diagnose heart failure. A special program processes ultrasound images, monitors the cardiac cycle and provides accurate measurements that help assess the risks of cardiovascular diseases. In 2023, the Food and Drug Administration (FDA) of the US called the invention of Ultromics for the detection of cardiac amyloidosis an unprecedented valuable innovation.
Bay Labs
This company creates AI-based tools for cardiology. They analyze heart images, ECG waveforms, and laboratory tests to detect cardiovascular diseases.
Oncology
These tools focus on pathology visualization, genomic data analysis, and personalized medicine predictive analytics to improve cancer diagnosis and treatment decisions.
Examples
Page and Ibex Medical Analytics are well-known predictive analytics in healthcare examples. Also, AsymMirai is a cancer prediction model that can predict breast cancer risk up to 5 years in advance.
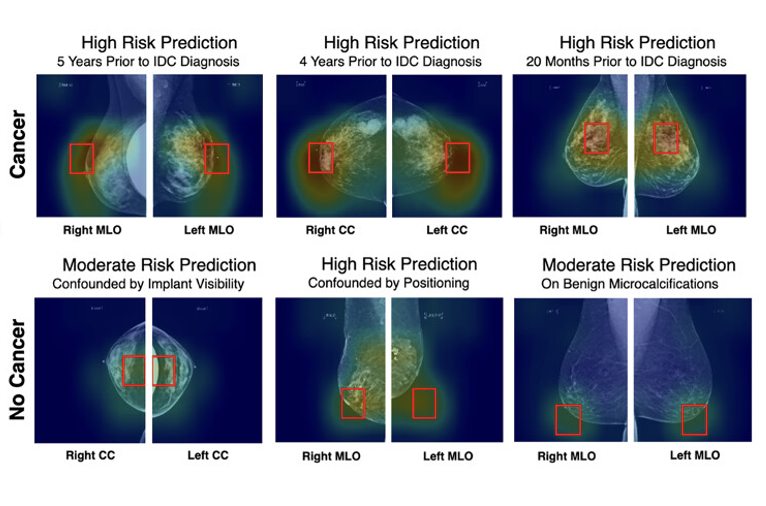
Radiology
AI tools in radiology use data mining to detect abnormalities in CT scans, X-rays, and other medical images and assist in risk stratification.
Zebra Medical Vision (Israel)
The company develops and supplies worldwide AI tools for radiology image analysis. Its AI models are used to detect a wide range of diseases, including cardiovascular diseases, lung diseases, liver diseases, and bone fractures. The system has been implemented in several hospitals, helping radiologists diagnose diseases quickly and accurately.
Ophthalmology
One of the benefits of AI in healthcare is that it helps diagnose retinal diseases by evaluating OCT, fundus images, and other eye scans.
IDx-DR and Eyenuk are predictive analytics in healthcare examples in this field.
These tools also integrate data from electronic health records to provide a comprehensive view of patient eye health.
Are you interested in healthcare mobile application development like for one of the abovementioned products? Then you’re in the right place.
Here’s what our clients say of Riseapps as of telemedicine app development company: “Riseapps has performed well and delivered tangible value. During the onset of the COVID-19 pandemic, they quickly built a telemedicine app. They communicate proactively to make the partnership as easy as possible.” — Coleman Warren, Director of Products, VieMed Healthcare
How predictive AI is used in healthcare
AI predictive analytics helps healthcare organizations in many ways. It allows for providing better and more proactive patient care, using data-driven insights to handle business tasks more efficiently, and cutting or avoiding unnecessary expenses to ensure higher profitability.
Providing better patient care
- Catching diseases earlier (think sepsis, specific cancers). Diagnosis using artificial intelligence can reduce the number of false positive and false negative results compared to radiologists. Ezra uses AI to analyze MRI scans and detect cancer early. AI is already able to diagnose lung cancer by analyzing large photographs of lung tissue. There are also AI developments for the diagnosis of skin cancer from photographs.
- Keeping high-risk patients healthier and out of the hospital (fewer readmissions). According to the National Library of Medicine the U.S. healthcare system spends about $52.4 billion a year on readmission. Predictive analytics in the healthcare sector makes it possible to identify patients with high probability of readmission, which allows doctors to allocate resources for follow-up and personalize discharge protocols.
- Tailoring treatments more effectively. Machine learning algorithms can process and analyze data more accurately than humans. Using AI to manage patient data and documentation electronically can minimize errors and improve data exchange capabilities.
Handling business efficiently
- Knowing staffing needs before the rush hits. AI systems optimize the work schedules of doctors and medical staff, and manage patient queues. This reduces waiting times and increases patient satisfaction.
- Getting ahead of operational bottlenecks (like ER wait times). AI predicts the number of patients requiring hospitalization or outpatient treatment. This helps to plan the work of medical institutions and avoid overloads.
- Optimizing the resources – beds, equipment, supplies. Thanks to digital analytic tools it became possible to optimize inventory management of medicines, medical equipment, and supplies.
Increasing the bottom line
- Cutting waste and unnecessary costs (prevention is cheaper than cure). Automation of administrative tasks and optimization of work processes can lead to significant cost savings for healthcare organizations. Reducing unnecessary face-to-face visits and switching to virtual consultations helps reduce the overhead associated with maintaining physical space.
More real-world use cases of AI predictive analytics in healthcare
Healthcare predictive analytics prognoses the likelihood of conditions such as diabetes or heart disease, enabling preventive care to take the lead in keeping the population healthier. The same analytics applied to the hospital management allow to predict patient flows, staff and resources requirements, ensuring efficient workflows that help more people while costing less.
Below are the examples of real-world applications that healthcare predictive analysis has made possible.
Improving patient outcomes and preventing complications
- Flagging patients likely to develop sepsis hours earlier than we could before.
- Identifying diabetic patients needing extra support before complications arise.
- Anticipating potential bad reactions to specific medications based on a patient’s profile, improving safety and patients outcomes.
Example:
Arizona State University (ASU) has developed a model that predicts how a patient’s immune system reacts to a new drug. The algorithm focuses on the functionality of MHC-1 proteins, on which the body’s ability to recognize foreign cells depends.
For example, a patient with hypertension experiences side effects from taking their current medication, hydrochlorothiazide. Severe dehydration and sometimes hypokaliemia are among the ailments.
Thanks to the machine model, it is possible to obtain results on the basis of which health care providers can transfer a patient to another class of antihypertensive drugs, for example, angiotensin II receptor blockers (ARBs). This drug is usually well tolerated and does not cause side effects such as hydrochlorothiazide.
- Helping doctors choose the most effective treatment path (e.g., for cancer) by predicting how an individual patient might respond.
- Flagging patients on general floors who show subtle signs of getting sicker, allowing for faster intervention before a code blue.
- Assisting radiologists by predicting which scans likely contain critical findings, helping them prioritize their workload for faster diagnoses.
Enhancing operational & financial efficiency
- Predicting patient no-shows so healthcare organizations can fill slots and optimize scheduling.
Example:
The King Faisal Specialist Hospital and Research Center (KFSHRC) in Riyadh has been trying for many years to deal with the problem of patient no-shows.
The board of Directors of the hospital decided to implement a forecasting system that, using machine learning, will determine the probability of patients not showing up for an appointment based on data from electronic medical records (EHR). This model was developed to reduce the number of absences among patients at risk and showed a fairly high level of accuracy. The researchers found that the number of patient absences in the past is a basic marker of future absences. Using the AI model, many such conclusions were drawn and a number of actions were planned that helped KFSHRC save on expensive resources and provide high-quality patient care.
- Forecasting ER admissions and surges to staff appropriately and manage patient flow better.
Example:
AI models can predict the number of hospital admissions by analyzing electronic health records, local events, and seasonal trends. These forecasts allow healthcare professionals to create a staffing plan, allocate resources (such as beds or equipment), and effectively monitor supply chains. For predictive analytics healthcare examples, the Cleveland Clinic has developed a readmission risk assessment tool to predict the likelihood of a patient’s readmission. This predictive analytics tool analyzes data from electronic medical records (EHRs) considering various factors, such as previous hospital visits (especially to the emergency department) or the type of hospitalization.
The score varies from 1 to 100. If the patient’s score is above 40, he falls into the 5% most likely candidates for readmission. This tool proved to be effective for healthcare organizations even during the COVID-19 pandemic. However, when diagnosing rarer infections it is less accurate.
- Pinpointing patients likely to be readmitted soon after discharge so organizations can intervene proactively with follow-up care.
- Predicting how long admitted patients will likely need to stay, improving bed management and discharge planning.
- Optimizing resource allocation by predicting demand for specific resources like blood products or expensive medications.
- Identifying insurance claims likely to be denied before submission, allowing finance teams to fix issues upfront and speed up payments.
- Forecasting when critical equipment (like MRI or CT scanners) might need maintenance before they break down, minimizing costly downtime.
What it takes to get started with AI predictive analytics?
So, how to use predictive analytics in healthcare, and more important – how to build it?
Creating a predictive analytics model requires a thorough, step-by-step plan.
The key steps for predictive modeling creation:
- Set your goal. Start by asking crucial questions. What task are you trying to solve? What new ideas or forecasts do you want to receive? Having a clear problem statement will help you with data collection, model selection, and effort verification. What specific problem do you want to predict?
- Get your data house in order. Collect and prepare the historical data necessary for your model. Find all possible data sources, both inside and outside your organization. Ensure that the data is clean, consistent, and complete. Use reliable data mining and data preparation methods to avoid errors or missing information.
- Build or partner. Choosing the right predictive analytics tool and learning it well are key steps in using predictive analytics in healthcare. You need to consider various statistical methods and machine learning algorithms, as well as identify risk factors. It helps to find the best model for your clinical problem and data.
- Integrate smartly. It is important to make sure that these tools help rather than hinder patient care quality. Overcoming the challenges of integrating the clinical workflow is key to making effective use of predictive analytics.
- Test, refine, repeat. For testing and verification, the data is divided into training and test sets. The model is trained on the training data. Then it is tested on the test data. This checks whether the model performs well on new data, and not just on those on which it was trained. Metrics such as accuracy, precision, recall, F1 score, and ROC AUC are used.
How to avoid hurdles with healthcare predictive analytics
AI in medicine promises to revolutionize diagnosis, treatment, and patient management. However, despite its enormous potential, the introduction of AI into medical practice and clinical decisions making faces a number of challenges:
- Data headaches. One of the main problems is the quality of the data. Getting clean, connected data in compliance with privacy (HIPAA) is paramount. AI requires huge amounts of data for training, but medical data is often incomplete, inaccurate, or not up to standard. For example, errors in patient records or differences in data formats can significantly reduce the effectiveness of AI algorithms. Accurate data is crucial for predicting health risks and ensuring the effectiveness of AI algorithms.
Good news! Experienced AI consultants and developers like the ones we have at Riseapps have overcome all these hurdles many times by now. Let’s save you the trouble and make a leading AI predictive analytics application for your healthcare organization.
➡️ See how Riseapps managed the large data volumes processing for PareIT medical summarization software
- Getting people on board. Clinicians need to trust the AI insights. The adoption of predictive analytics in health care by the medical community is usually slow. Doctors and medical staff may be skeptical of AI, fearing that it will replace their work or lead to mistakes. Training and advanced training of medical personnel are key factors for the successful implementation of AI.
- Being transparent about how it works – avoiding the “black box” fear. AI systems often operate as “black boxes” where decision-making processes remain unclear even to developers. In medicine, where accuracy and validity of decisions are vital, this is a serious problem. For example, if an AI system recommends a certain treatment, but cannot explain why it made such a choice, this may cause distrust on the part of medical professionals and patients.
- The tech & cost factor. Predictive modeling requires investment and integration with the current healthcare system. This includes not only the development and training of models, but also the purchase of necessary equipment, staff training, and healthcare IT system support.
- Regulations & responsibility. Who is responsible for the mistakes made by AI? This issue remains open and requires clear legal regulation. In the case of an incorrect diagnosis or treatment, the responsibility may lie with AI developers, medical institutions, or even the doctors themselves.
How Risapps can help leverage AI-powered predictive analytics
When choosing the right partner for your clinic, consider the focus of your company. The fact is that so far there is no single tool in the world that would solve all problems when it comes to artificial intelligence technologies in healthcare.
If you want to optimize critical business indicators – supply chains, sales, customer service, or staff efficiency – healthcare predictive analytics will help you do this.
Predictive analytics tools help you better understand your audience and business processes so that you can determine which actions bring the most benefit to them and your organization and drive actionable insights for your business.
What’s more important, it increases effectiveness and productivity for your doctors and stakeholders.
If you are looking for an AI-based platform for predictive analytics in healthcare use cases, Riseapps is a custom software development company that can build one for you.
Specializing in telemedicine app development, AI strategy consulting and generative AI development, the company offers a range of services, including mobile and web development, cross-platform solutions, user interface design, and software testing.
Riseapps provides customized AI-based solutions certified according to ISO 27001 and ISO 9001 standards, as well as meeting the requirements of HITECH, GDPR, HIPAA.
FAQ
How does AI help optimize patient care?
AI can transform healthcare by making diagnoses and predicting disease progression faster and more accurate. It can also help with treatment planning, disease prediction, and decision support for doctors.
What is the difference between AI and AI predictive analytics?
Both AI and predictive analytics healthcare are used in healthcare to improve patient outcomes. However, AI mimics human intelligence by performing tasks such as data processing, pattern recognition, and decision-making. Predictive analytics uses historical data and statistical modeling to predict future outcomes. While AI performs a broader range of tasks, predictive analytics specifically examines probabilities and trends to support proactive decision-making in the healthcare sector.
How does AI improve the diagnosis of diseases?
AI analyzes big medical data, including images, laboratory results, and patient medical history, at a speed and scale beyond human capabilities. Thus, doctors can identify patterns, anomalies, and chronic diseases more accurately. This helps to identify high risk patients, make more accurate diagnoses and optimize treatment plans.
What is the future of AI predictive analytics in medicine?
Among the trends is the increased use of AI in personalized medicine, where treatments are tailored to individual genetic profiles. In addition, AI-based predictive models will play a crucial role in preventive medicine and population health management, helping to detect diseases before symptoms appear.
Contact Us
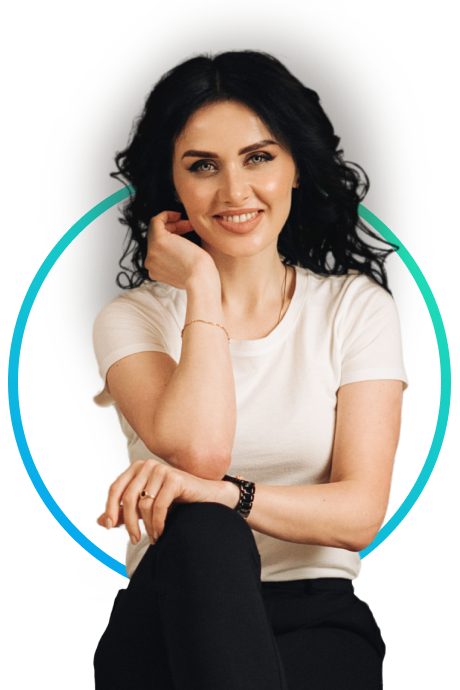