Machine Learning in Healthcare Industry
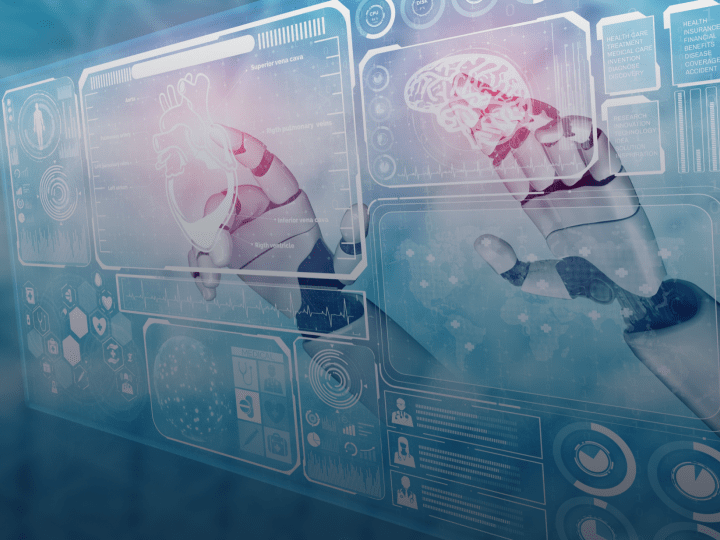
Technology benefits the healthcare industry significantly. Embracing EHR, the Internet of Things, telehealth, and other information technologies is changing how we get medical treatment. Machine learning is a subset of artificial intelligence that is developing rapidly nowadays. Artificial intelligence in the healthcare market is expected to reach USD 40.2 billion by 2026, at a CAGR of 49.7%.
ML already simplifies disease diagnosis and helps physicians draw up treatment plans more precisely. Its algorithms process vast amounts of patients’ data to make reasonable conclusions faster and more accurately than the human brain.
Although machine learning technologies are far from completely replacing humans, they greatly simplify physicians’ work and help make smart decisions. Let’s take a closer look at how machine learning is improving the healthcare industry.
The examples of machine learning applications in healthcare
Machine learning is a branch of computer science that works on data and algorithms to imitate how the human brain learns, continuously improving its accuracy. The field of applications in the healthcare industry is quite broad.
Apart from healthcare, machine learning is used in different industries such as marketing, finance, logistics, etc., with excellent results. Big corporations use advanced algorithms to predict what kind of goods we are likely to buy and what content we’d prefer to consume. People can apply the same algorithms for the healthcare industry to bring valuable outcomes for society.
Keeping health records
In the early 90s, when personal computers and the Internet became more accessible, hospitals actively began to implement electronic medical record software. According to the Centers for Disease Control and Prevention, 85% of office-based physiciansuse an EMR/EHR system.
Embracing technology to keep health records made medical information more accessible. Still, there’s a problem with how to collect data effectively. Machine learning can address this problem by offering tools for fast data processing. Such technologies as natural language processing and optical character recognition facilitate data collection and accelerate administrative workflow.
Take Ciox as an example. Ciox is a healthcare data management software trusted by over 700 thousand providers from the US. The company developed a data platform called HealthSource to provide data interoperability. AI and ML tools simplify health information flow and help manage unstructured records and access data nationwide. Besides that, the platform offers natural language processing and handwriting recognition tools to enhance data collection.
Increasing treatment protocol accuracy
Treatment planning often requires a lot of time and knowledge of medical specialists. Developing a treatment plan is highly complex when we talk about cancer therapy. Depending on each patient’s body type and tumor peculiarities, therapists should develop balanced treatment procedures to deliver precise portions of radiation to the tumor and minimize irradiation to healthy organs.
Machine learning algorithms can analyze patients’ medical information and create personalized treatment plans based on previous successful treatment plans databases. The study reveals that machine learning-based treatment plans were preferred to human-made ones and were used in 88% of prostate cancer radiation therapy cases.
RaySearch Laboratories is a leading software developing company for cancer radiation therapy trusted by over 2,600 clinics worldwide. One of their products, RayStation, is a treatment plan optimization solution. The software has machine learning algorithms in its core which consider patient geometry, doses, and treatment history to provide optimized personal treatment plans. The system also includes accessibility as it doesn’t keep personal data, so clinics can share treatment experiences and improve therapy practice.
Processing medical images
Medical imaging is getting pictures of the human body to apply to the diagnosis and treatment of patients. Therapists also use imaging to track ongoing health issues and adjusting treatment plans.
Modern medical imaging systems keep thousands of images with valuable patients’ information. However, extracting this information requires time and effort. Image analysis algorithms can process visual data to help practitioners make reasonable medical decisions and improve their workflow.
Let’s take PathAI as an example of how machine learning can improve medical image analysis. The PathAI platform detects different types of cells on the image to help pathologists recognize malicious cells.
Machine learning algorithms help detect cancer from tissue samples images more accurately and faster than a human. Moreover, algorithms can learn from the previous outcomes to help pathologists provide patients with the most helpful diagnosis.
Developing new treatments and medicine
The traditional drug development process is complex. It requires a lot of time and financing to put the drug to market. It could take more than ten years and over USD 2 billion to develop a new medicine.
Machine learning can assist chemists and pharmacists in boosting the drug discovery pathway. ML algorithms apply to each stage of drug discovery, from target validation to digital data processing in clinical trials. The main goal of ML in the pharmaceutical industry is to improve processes and outcomes. For example, Pfizer’s pharmaceutical company used Al technologies to accelerate clinical trials when developing the Covid-19 vaccine. The algorithms managed to clean up patients’ data in less than 24 hours when it usually takes up to 30 days with manual datasets analysis. The ML tool also provided quality data throughout the trial with little human intervention.
Improving analytics
Analytics in healthcare focuses on improving care experience. Patient data measurement and analytics facilitate decision-making by providing evidence to support medical insights. There are few stages of analytics capabilities. Descriptive and diagnostic analytics are traditional methods that work with past data to create statistical reports such as a percentage of patients sick with flu compared to last and previous months.
The next analytics stages are predictive, prescriptive, and cognitive analytics. These methods require advanced computational algorithms to perform forecasting, real-time suggestions, and automated decision-making. Machine learning techniques provide the healthcare industry with insightful information and help to make intelligent decisions. Analytic outcomes, when decoded right, can enhance the treatment process, speed up recovery, foreseen relapses, save costs, and more.
AI-based analytics is developing rapidly. Such companies as ConcertAI use real-world data and machine learning models to optimize patient care and promote recovery. ConcertAI provides on-demand access to real-world data repositories and AI-driven oncology solutions to improve cancer treatment. Recently, the company started a collaboration with FDAto bring real-world evidence to regulatory decisions.
Providing data security
Technology integration in the healthcare industry has led to a growth of sensitive data. Patients’ records, personal information, diagnostic reports, test results are excellent targets for manipulation by cybercriminals. Dealing with data breaches’ consequences is costly and time-consuming. According to the Bitglass healthcare breach report, the average cost per breached record in 2020 was $499. Considering 26.4 million records were hacked, healthcare organizations spent $13.2 billion on data breaches. That’s why protecting patients’ data is crucial for the healthcare industry.
Machine learning algorithms can improve fraud detection and prevent cyberattacks using predictive analytics. Security Information and Event Management (SIEM) software analyzes real-time security alerts from a healthcare organization network to provide security reports for compliance purposes. Powered with machine learning models, SIEMs could improve attack detection. The software tracks users’ activities to understand the typical usage patterns and then distinguishes suspicious activities within the system. If the system discerns the user behavior as unusual, it can flag the action as a fraud or shut the user out from the network.
Darktrace is software that uses AI technologies to detect cybersecurity threats within healthcare providers’ cloud and corporate networks. The software learns from ‘the patterns of life’ to understand normal user behavior and reveal malicious activities. One of the case studies of Darktrace claims that the healthcare organization got positive ROI thanks to automation that offers the software. Cybersecurity is a massive concern for many organizations nowadays, and machine learning can handle cyber threats more precisely than any high-quality security analyst.
Personalizing patient care
Personalized medicine is based on diagnostic tests to determine the best medical treatment for each individual. Physicians consider a patient’s clinical history, circumstances, and risk factors to develop a unique treatment plan or prevent disease relapse.
Personalized medicine is gaining momentum due to improved knowledge about diseases and new analytics approaches powered by AI technologies. As the volumes of medical data rise, there are opportunities for machine learning to bring benefits to healthcare:
-
- Healthcare organizations can reduce costs with the automation of diagnosis and prescription processes.
-
- Personalized medical services increase patient satisfaction.
-
- Disease diagnosis at early stages lowers mortality rates.
-
- Real-time treatment powered with AI could save lives.
There are a lot of use cases of machine learning for personalized patient care. For example, GNS Healthcare enhances machine learning algorithms to capture patients’ drug responses and suggest the most effective treatment. In 2019, the company raised $23 million from drug companies and medical insurance companies.
The future potential of Machine Learning in Healthcare
Machine learning has the potential to transform the ways physicians deliver medical care. Automation and predictive analytics will enable caregivers to refocus their efforts on improving patient care rather than dealing with mundane tasks. AI-based technologies could take over different practitioners’ duties, from administrative functions to fast and precise diagnosis to remote real-time treatment. This shifting paradigm will transform the approaches to medical education. Future practitioners will require advanced skills of working with data and innovations, continuous learning, and operating with knowledge from different disciplines.
The AI development in healthcare is moving towards hybrid models that can support clinicians in diagnosing diseases, drawing up treatment plans, monitoring patients, and analyzing risk factors while leaving humans the opportunity to make responsible decisions. According to the Intel survey, only 37% of healthcare decision-makers already use AI tools. Many operational processes in medicine still need to be improved, and artificial intelligence technologies, including machine learning, can contribute to the healthcare industry. Therefore, the development of AI-powered healthcare applications is in high demand nowadays. We are moving towards a high-tech society in which medicine is acquiring new tools for interacting with patient data to treat people more effectively.
How Riseapps can help you integrate ML in a healthcare app
Riseapps’ healthcare app development team has expertise in integrating machine learning algorithms into healthcare applications and developing medical software. Our projects included developing a remote patient monitoring tool that collects, stores, and manages patients’ sensitive data following HIPAA requirements. The app has sophisticated algorithms in its core that are executed according to precise instructions to track changes in patients’ data and inform practitioners in time. As a result, we’ve provided a risk-free solution that doesn’t miss a single case.
Wrapping up
Machine learning in the healthcare industry serves as a booster of medical outcomes. Computer algorithms can process constantly increasing volumes of data to carve insightful ideas on improving patient care, diagnosis, drug discovery, and more. Technology helps caregivers let go of routine tasks and focus on helping people. Such severe diseases as cancer can be cured more precisely with the help of AI technologies. No doubts the role of machine learning in healthcare will only increase in the foreseeable future.
FAQs
What are the benefits of machine learning in healthcare?
Machine learning helps the healthcare industry automate routine tasks such as drawing up treatment plans, tracking changes in patients’ clinical data, etc. Moreover, ML algorithms provide fast and precise data analysis for drug discovery and predictive medicine. Also, ML tools improve disease identification and medical images analysis. With the help of machine learning, healthcare organizations free up more time for taking care of patients rather than dealing with data management.
Is machine learning the future of healthcare?
Machine learning has the potential to transform the ways physicians deliver medical care. ML facilitates data management and helps get valuable outcomes in healthcare, such as lowering mortality rates and accurate cancer diagnosis and treatment. In the foreseeable future, the adoption of machine learning within healthcare organizations will only increase.
What are examplesof machine learning in healthcare
Machine learning is used in healthcare to handle different kinds of tasks. For example, Machine learning can assist chemists and pharmacists to boost the drug discovery pathway. ML algorithms apply to each stage of drug discovery, from target validation to digital data processing in clinical trials. The other way to engage ML for medical purposes is to use predictive analytics to identify patients’ health changes early and prevent diseases from relapsing.
Want to boost your healthcare app with machine learning algorithms? Riseapps can help you with machine learning integration for your custom solution.
FAQ
What are the benefits of machine learning in healthcare?
Machine learning helps the healthcare industry automate routine tasks such as drawing up treatment plans, tracking changes in patients' clinical data, etc. Moreover, ML algorithms provide fast and precise data analysis for drug discovery and predictive medicine. Also, ML tools improve disease identification and medical images analysis. With the help of machine learning, healthcare organizations free up more time for taking care of patients rather than dealing with data management.
Is machine learning the future of healthcare?
Machine learning has the potential to transform the ways physicians deliver medical care. ML facilitates data management and helps get valuable outcomes in healthcare, such as lowering mortality rates and accurate cancer diagnosis and treatment. In the foreseeable future, the adoption of machine learning within healthcare organizations will only increase.
What are examplesof machine learning in healthcare
Machine learning is used in healthcare to handle different kinds of tasks. For example, Machine learning can assist chemists and pharmacists to boost the drug discovery pathway. ML algorithms apply to each stage of drug discovery, from target validation to digital data processing in clinical trials. The other way to engage ML for medical purposes is to use predictive analytics to identify patients' health changes early and prevent diseases from relapsing.
Want to boost your healthcare app with machine learning algorithms? Riseapps can help you with machine learning integration for your custom solution.
Contact Us
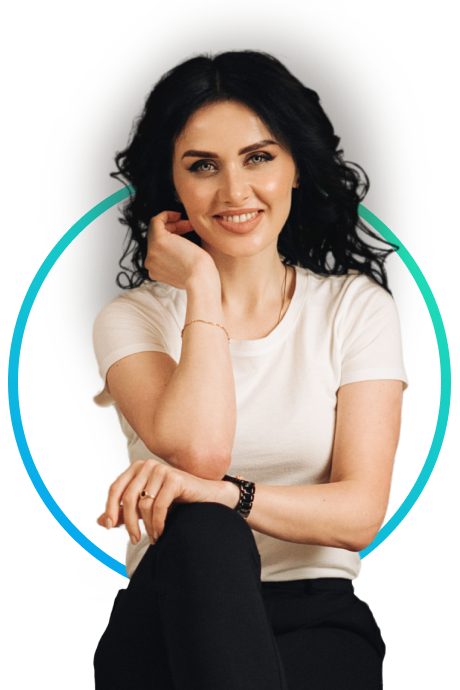